Embracing AI in Endodontics: Pioneering Detection of External Cervical Resorption
By Ali Nosrat, DDS, MS, MDS, and Hossein Mohammad-Rahimi, DDS
The Dawn of AI in Endodontics
Artificial intelligence’s (AI) capability to learn from data without explicit programming allows it to uncover intricate patterns and relationships within vast datasets, surpassing the diagnostic accuracy achievable by humans alone.1 This machine learning process is particularly beneficial in endodontics, where the accurate interpretation of diagnostic images plays a crucial role in the success of treatment. By analyzing radiographs and other diagnostic images, AI algorithms can identify subtle signs of disease such as periapical lesions2, vertical root fractures3, and variations in canal anatomy4 that might be overlooked by the human eye.
The implications of AI in endodontics extend beyond diagnostics. AI can assist in the identification of case difficulty and referral decisions5, predict the risk of post-treatment complications6, and enhance the learning and training of endodontic professionals in various ways.7 Moreover, the automation of routine tasks by AI allows clinicians to dedicate more time to complex procedures and patient interaction, enhancing the overall efficiency of the clinical workflow. As AI technology continues to evolve, its integration into endodontic practice is expected to become more prevalent, leading to more predictable, efficient, and personalized patient care.
Finally, the recent rise of AI chatbots presents exciting possibilities for the field of endodontics.8 Imagine a world where patients can access a 24/7 resource for basic endodontic questions, appointment scheduling, and post-operative care instructions. AI chatbots on practice websites can answer frequently asked questions about root canal procedures, alleviate pre-appointment anxiety, and even collect pre-operative health data. This not only frees up valuable staff time but also empowers patients with readily available information, fostering a more informed and engaged experience throughout their endodontic journey.
Challenges and Considerations
The potential of AI in revolutionizing endodontic practice is immense. However, the integration of AI into endodontics is not without its challenges. These obstacles are multifaceted, encompassing technical, ethical, and regulatory domains. These include:
- Data Availability and Quality: The cornerstone of effective AI models lies in the availability of large, well-labeled datasets. In endodontics, specifically for rare conditions such as external cervical resorption (ECR), obtaining enough labeled data poses significant challenges. This process is not only time-consuming but also costly. Self-Supervised Learning (SSL) models9 or active learning10 models were explored to mitigate this challenge by reducing reliance on extensive labeled datasets.
- Interpretability and Trust: The ‘black box’ nature of AI models raises interpretability concerns. For clinicians to trust and effectively use AI in their practice, they need to understand how decisions are made by these models. This transparency is crucial for integrating AI outputs into clinical decision-making processes. Trust in AI can be bolstered by developing models that not only excel in performance but are also capable of explaining their reasoning in terms that clinicians can understand.11
- Regulatory and Ethical Considerations: The use of AI in healthcare, including endodontics, introduces complex regulatory and ethical considerations. Ensuring patient safety and privacy, obtaining informed consent for the use of data, and navigating the regulatory approvals for clinical use are paramount. The ethical implications of AI decisions, especially in cases of diagnostic uncertainty or errors, must be addressed comprehensively to ensure that patient care remains the foremost priority.12
In recent years, the field of endodontic research has witnessed revolutionary changes through the integration of AI in diagnosing complex dental conditions. This evolution has marked a significant shift from traditional diagnostic methods, introducing efficiency and precision that can result in a significant change in the future of “diagnostic methods” in our field. Inspired by our recent study titled “Artificial Intelligence for Detection of External Cervical Resorption Using Label-Efficient Self-Supervised Learning Method” 9, this article explores the transformative impact of AI on the future of endodontics, focusing on the innovative detection of ECR.
A Focus on External Cervical Resorption
ECR is an invasive type of resorption originating from periodontium that can progress through a non-linear pattern at a slow pace.13 Early and accurate diagnosis of ECR is crucial for successful management/treatment.14,15 Traditionally, ECR is diagnosed through radiographic examination. However, differentiating ECR from other pathologies such as caries on radiographs can be challenging. Its asymptomatic nature and the intricacies involved in its detection have made it a focal point for applying AI technologies.
ECR is a relatively uncommon condition so acquiring a high-quality and large annotated dataset is difficult. In our recent study we explored the application of label-efficient SSL in detecting ECR, a method that drastically reduces the reliance on large, annotated datasets.9 The study implemented nine state-of-the-art contrastive SSL models as well as seven baseline models based on convolutional neural networks (CNNs) which are specifically designed for the analysis of images. The aim of the study was to assess AI’s capability to detect ECR and differentiate it from dental caries.
Our results highlighted the DINO model as one of the innovative solutions, demonstrating highest test set accuracy of 84.09% in correctly labeling three Regions of Interest: ECR, Caries, and healthy tooth (without ECR or caries). However, the study also revealed specific limitations within the DINO model, as shown in Figure-1. For instance, in cases where the resorption was at a very early stage or presented with atypical manifestations, the DINO model faced challenges in accurately distinguishing ECR from dental caries or healthy teeth with no ECR or caries.
This not only highlights AI’s potential in enhancing diagnostic accuracy but also underscores the importance of innovative approaches in overcoming the challenges of limited annotated datasets in dental imaging.
Conclusion
The results of this study presented a significant leap forward in endodontic diagnostics. The advent of AI in endodontics, particularly through the innovative application of SSL models for detection of ECR, marks a significant milestone in dental diagnostics. As we continue to explore and integrate AI technologies in dentistry, the potential for improved patient outcomes, enhanced diagnostic accuracy, and streamlined treatment planning becomes increasingly evident. The future of endodontics is bright, with AI leading the way towards a new era of dental care characterized by precision, efficiency, and innovation.
Imagine a future where AI algorithms analyze radiographs and CBCT scans, not only identifying complex root canal anatomy and potential procedural challenges but also flagging defects such as ECR that are overlooked by the clinician. This advanced diagnostic support could allow endodontists to tailor strategies for each unique case.
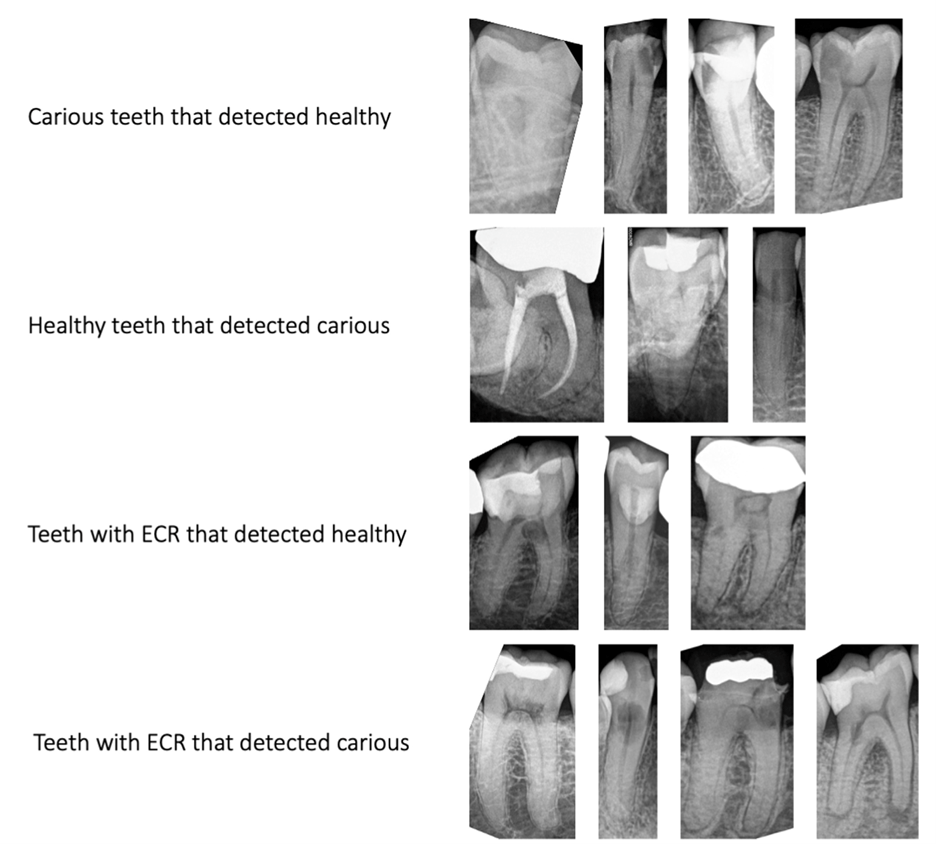
Figure 1. Samples of DINO misclassifications
References
1 Mohammad-Rahimi, H., Rokhshad, R., Bencharit, S., Krois, J. & Schwendicke, F. Deep learning: A primer for dentists and dental researchers. Journal of Dentistry 130, 104430, doi:https://doi.org/10.1016/j.jdent.2023.104430 (2023).
2 Sadr, S. et al. Deep Learning for Detection of Periapical Radiolucent Lesions: A Systematic Review and Meta-analysis of Diagnostic Test Accuracy. Journal of Endodontics 49, 248-261.e243, doi:https://doi.org/10.1016/j.joen.2022.12.007 (2023).
3 Fukuda, M. et al. Evaluation of an artificial intelligence system for detecting vertical root fracture on panoramic radiography. Oral Radiology 36, 337-343, doi:10.1007/s11282-019-00409-x (2020).
4 Sherwood, A. A. et al. A Deep Learning Approach to Segment and Classify C-Shaped Canal Morphologies in Mandibular Second Molars Using Cone-beam Computed Tomography. Journal of Endodontics 47, 1907-1916, doi:https://doi.org/10.1016/j.joen.2021.09.009 (2021).
5 Mallishery, S., Chhatpar, P., Banga, K. S., Shah, T. & Gupta, P. The precision of case difficulty and referral decisions: an innovative automated approach. Clinical Oral Investigations 24, 1909-1915, doi:10.1007/s00784-019-03050-4 (2020).
6 Qu, Y. et al. Machine learning models for prognosis prediction in endodontic microsurgery. Journal of Dentistry 118, 103947, doi:https://doi.org/10.1016/j.jdent.2022.103947 (2022).
7 Aminoshariae, A. et al. Artificial Intelligence in Endodontic Education. Journal of Endodontics, doi:https://doi.org/10.1016/j.joen.2024.02.011 (2024).
8 Mohammad-Rahimi, H. et al. Validity and reliability of artificial intelligence chatbots as public sources of information on endodontics. International Endodontic Journal 57, 305-314, doi:https://doi.org/10.1111/iej.14014 (2024).
9 Mohammad-Rahimi, H. et al. Artificial Intelligence for Detection of External Cervical Resorption Using Label-Efficient Self-Supervised Learning Method. Journal of Endodontics 50, 144-153.e142, doi:https://doi.org/10.1016/j.joen.2023.11.004 (2024).
10 Huang, J. et al. Uncertainty-based Active Learning by Bayesian U-Net for Multi-label Cone-beam CT Segmentation. Journal of Endodontics 50, 220-228, doi:https://doi.org/10.1016/j.joen.2023.11.002 (2024).
11 Ma, J. et al. Towards Trustworthy AI in Dentistry. Journal of Dental Research 101, 1263-1268, doi:10.1177/00220345221106086 (2022).
12 Rokhshad, R. et al. Ethical considerations on artificial intelligence in dentistry: A framework and checklist. Journal of Dentistry 135, 104593, doi:https://doi.org/10.1016/j.jdent.2023.104593 (2023).
13 Nosrat, A. et al. External Cervical Resorption: A Volumetric Analysis on Evolution of Defects over Time. Journal of Endodontics 49, 36-44, doi:https://doi.org/10.1016/j.joen.2022.10.010 (2023).
14 Mavridou, A. M. et al. Understanding External Cervical Resorption in Vital Teeth. J Endod 42, 1737-1751, doi:10.1016/j.joen.2016.06.007 (2016).
15 Patel, S., Foschi, F., Condon, R., Pimentel, T. & Bhuva, B. External cervical resorption: part 2 – management. Int Endod J 51, 1224-1238, doi:10.1111/iej.12946 (2018).